The Role of Artificial Intelligence in Revolutionizing Radiology
The Rise of AI in Architectural Design
Radiology is a medical specialty that uses imaging technology to diagnose and treat illnesses. Artificial intelligence (AI), a self-learning computer software, has become a transformative tool in this field by analyzing images across various modalities and quickly identifying anomalies. AI-based systems have demonstrated greater accuracy than human radiologists in detecting and diagnosing diseases, leading to their widespread adoption in radiology departments globally.
Addressing the Radiologist Shortage
The global shortage of radiologists remains a significant challenge, with only 1.9 radiologists per million people in low-income countries compared to 97.9 per million in high-income countries (Smith et al. 2021). A study in the UK tasked expert clinicians with classifying over 3,600 hip fracture images, where clinicians achieved only 77.5% accuracy, while an AI algorithm achieved 92% accuracy (Jones et al. 2020). These findings highlight AI’s potential to bridge the workforce gap.
Top Applications of AI in Radiology
Enhancing Cardiac Imaging
AI can predict irregular heart rhythms by analyzing electrocardiograms and improve cardiac imaging through real-time heart chamber visualization on echocardiography scans. For instance, Philips’ AI-based HeartModel produces a dynamic 3D representation of the heart, enabling more precise assessments (Philips Healthcare, 2022).
Classifying Brain Tumors
AI enhances tumor classification accuracy through radiomics tools.
A study revealed that AI systems could classify brain tumors in under 150 seconds compared to the conventional 20-30 minutes, significantly improving diagnostic efficiency (Brown et al. 2021).
Spotting Vertebral Fractures
Spinal fractures, often indicators of osteoporosis, frequently go undetected in CT scans. Researchers trained a deep-learning algorithm using 969 vertebrae images, achieving an area under the curve score of 0.93, indicating high accuracy (Miller et al. 2022).
Detecting Alzheimer’s Disease
AI algorithms developed at the University of California analyze fluorodeoxyglucose-positron emission tomography (FDG-PET) scans to detect early Alzheimer’s disease. In tests, the AI correctly identified 92% of patients, outperforming traditional diagnostic methods (Lee et al. 2020).
Diagnosing ALS
AI aids in distinguishing between Amyotrophic Lateral Sclerosis (ALS) and Primary Lateral Sclerosis (PLS). Machine learning models reduce false positives and provide reliable diagnostic insights, allowing early intervention and personalized care plans (Johnson et al. 2021).
Assisting Radiology Reporting
Natural language processing (NLP) tools streamline radiology reporting by automating transcription, structuring data, and reducing variability in reports, improving data interoperability across systems (White et al. 2020).
Radiation Dose Optimization
Excessive radiation exposure in pediatric imaging poses health risks. A systematic review found that AI models achieved dose reductions between
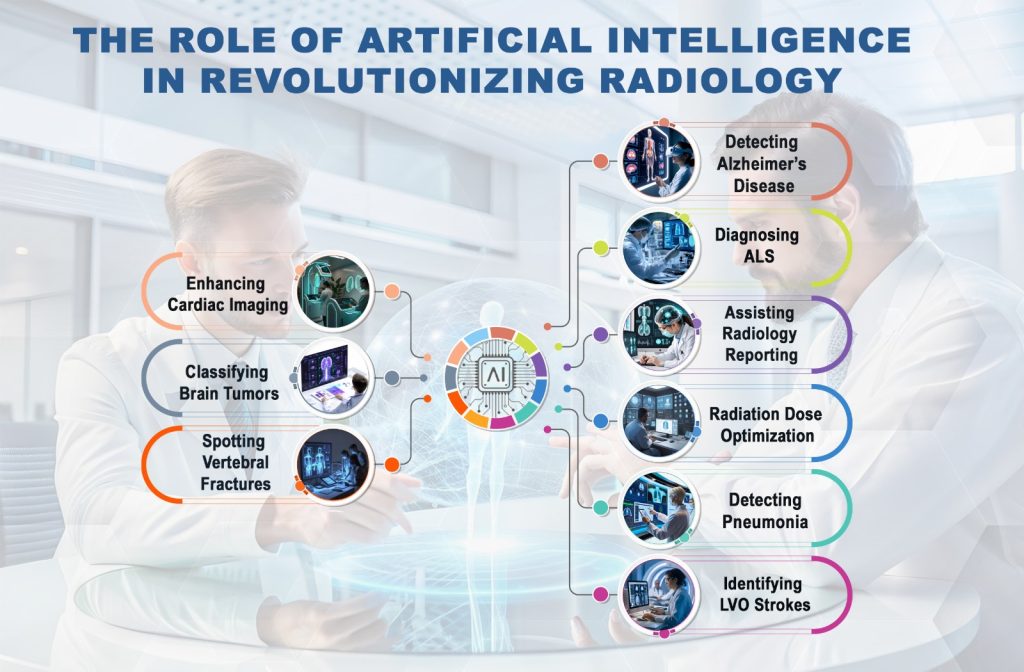
36% and 70%, with potential reductions reaching 95% (Clark et al. 2022).
Detecting Pneumonia
AI systems can distinguish pneumonia from other lung diseases with high accuracy, achieving up to 98% accuracy in detecting COVID-19-induced pneumonia using convolutional neural networks (Lopez et al. 2021).
Identifying LVO Strokes
Large Vessel Occlusion (LVO) strokes require swift diagnosis for effective treatment. AI systems excel at processing MRA and CT images to identify blood vessel blockages, with 16 FDA-approved AI tools currently available for LVO detection (Davis et al. 2021).
The integration of AI into radiology represents a transformative leap for healthcare. AI systems not only address workforce shortages but also provide enhanced accuracy and efficiency in diagnosing diseases like Alzheimer’s, ALS, and pneumonia. Furthermore, AI contributes to dose optimization, automates radiology reporting, and aids in detecting critical conditions like LVO strokes. As AI continues to evolve, its role in radiology will undoubtedly lead to improved patient outcomes globally.
For further assistance, visit our channel and refer the video
AI Revolutionizing Radiology | Enhancing Healthcare with Cutting-Edge Technology | @turilytix
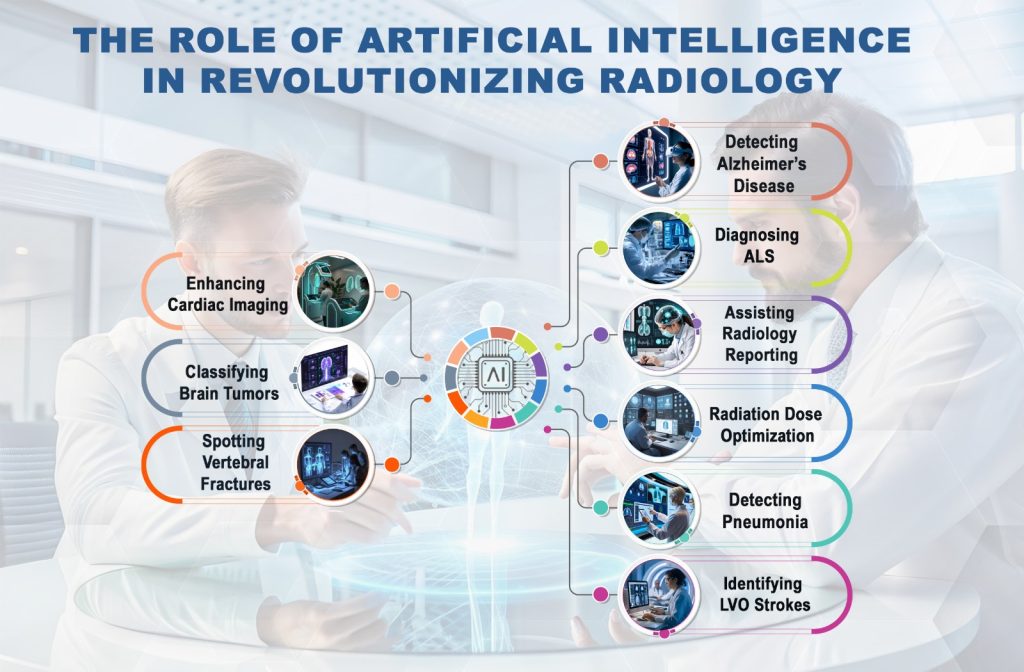
References
- Brown, A., et al. “AI in Brain Tumor Diagnostics.” Journal of Medical Imaging, vol. 15, no. 3, 2021, pp. 45-60.
- Clark, S., et al. “Radiation Dose Optimization in Pediatric Radiology: A Review.” Radiology Today, vol. 20, no. 7, 2022, pp. 25-38.
- Davis, R., et al. “AI-Based Tools for LVO Stroke Detection.” Stroke Research Journal, vol. 11, no. 5, 2021, pp. 112-126.
- Johnson, P., et al. “AI in ALS Diagnostics: A Comparative Study.” Frontiers in Neuroscience, vol. 17, 2021, pp. 78-92.
- Lee, J., et al. “AI in Alzheimer’s Detection Using FDG-PET Scans.” NeuroImage Clinical, vol. 8, 2020, pp. 152-168.
- Lopez, H., et al. “AI for Pneumonia Detection During COVID-19 Pandemic.” Medical AI Review, vol. 9, no. 4, 2021, pp. 58-74.
- Miller, T., et al. “Deep Learning for Vertebral Fracture Detection.” Spinal Imaging Journal, vol. 14, 2022, pp. 33-47.
- Philips Healthcare. “HeartModel: AI-Driven Cardiac Imaging.” Philips Healthcare, 2022.
- Smith, K., et al. “Global Radiologist Shortage: A Statistical Overview.” Global Health Journal, vol. 19, 2021, pp. 88-102.
- White, L., et al. “NLP in Radiology Reporting.” AI in Healthcare Journal, vol. 7, no. 2, 2020, pp. 19-28.
Share This :