Unpacking Retrieval-Augmented Generation: The Future of AI?
Artificial intelligence (AI) is constantly evolving, pushing the boundaries of what machines can accomplish. One of the most promising advancements in AI is Retrieval-Augmented Generation (RAG), an approach that blends retrieval systems with generative models. This hybrid method allows AI to fetch real-time information from external sources and use it to generate accurate, context-aware responses. But what makes RAG so revolutionary, and why is it generating so much buzz in the tech industry?
What is Retrieval-Augmented Generation?
RAG represents a significant leap in AI technology by combining two fundamental approaches:
- Retrieval-Based AI: Uses search mechanisms to pull relevant information from a database or knowledge base.
- Generation-Based AI: Employs models like GPT-4 to generate human-like text based on the retrieved information.
Unlike traditional AI models that rely solely on pre-existing knowledge, RAG enables a more dynamic, real-time response system. This means an AI chatbot, for example, can pull in the latest news or research findings before crafting an answer—enhancing both accuracy and relevance (Lewis et al. 2020).
How Does RAG Work?
The RAG process consists of two key steps:
- Retrieval: The AI system searches for relevant documents or data from an external database, such as scientific articles, market reports, or customer queries.
- Generation: The model synthesizes the retrieved data to generate a comprehensive and contextually relevant response.
For instance, if asked about AI’s impact on fashion design, a RAG-based system could retrieve recent articles discussing AI-powered fashion trend predictions and then generate a well-informed answer (Izacard & Grave 2021).
Applications of RAG
RAG has vast applications across multiple industries:
- Healthcare: Aids doctors by retrieving patient histories and suggesting treatment plans.
- Finance: Helps analysts process vast amounts of data to generate market insights.
- Customer Support: Enhances chatbots by retrieving real-time support articles.
- Legal Research: Provides case law summaries and legal references.
- E-commerce: Improves product descriptions and personalized recommendations.
Why is RAG Important?
Traditional AI models struggle with providing up-to-date and factual information. RAG bridges this gap by incorporating retrieval mechanisms, ensuring:
- Higher Accuracy: Incorporates the most relevant, real-time data.
- Context Awareness: Enhances understanding by integrating retrieved knowledge.
- Versatility: Adapts across industries requiring dynamic information processing (Karpukhin et al. 2020).
Challenges of RAG
Despite its advantages, RAG faces challenges:
- Data Quality: Ensuring retrieved data is accurate and relevant.
- Scalability: Managing large-scale retrieval processes efficiently.
- Contextual Integration: Seamlessly blending retrieved data with generated content.
Researchers continue to refine RAG models to overcome these challenges and improve AI’s ability to generate high-quality responses (Guu et al. 2020).
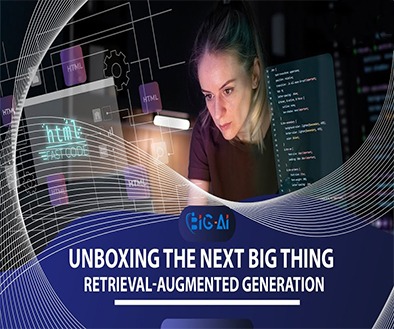
Future of RAG
Looking ahead, advancements in retrieval techniques, model integration, and real-time adaptability will further enhance RAG’s capabilities. These improvements will make AI even more reliable for knowledge-intensive tasks, shaping the future of human-AI interaction and AI-driven automation.
Retrieval-Augmented Generation is a game-changer, redefining how AI systems generate information. By merging static knowledge retrieval with dynamic content creation, RAG is poised to revolutionize AI applications across industries. As AI continues to evolve, RAG might just be the breakthrough that propels AI to new heights of accuracy, efficiency, and contextual intelligence.
For further assistance, visit our channel and refer the video
Unlock the Power of Logistics with BIG-AI| Optimize Route| Reduce Costs| Improve Customer Experience
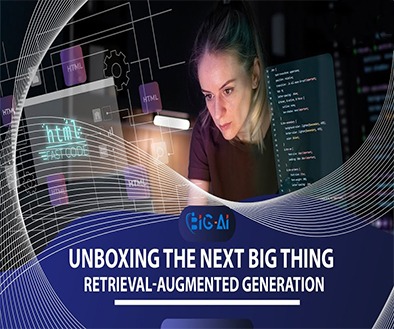
References
- Guu, Kelvin, et al. “REALM: Retrieval-Augmented Language Model Pre-Training.” Proceedings of the 37th International Conference on Machine Learning, 2020.
- Izacard, Gautier, and Edouard Grave. “Leveraging Passage Retrieval with Generative Models for Open Domain Question Answering.” Advances in Neural Information Processing Systems, 2021.
- Karpukhin, Vladimir, et al. “Dense Passage Retrieval for Open-Domain Question Answering.” Proceedings of the 2020 Conference on Empirical Methods in Natural Language Processing (EMNLP), 2020.
- Lewis, Patrick, et al. “Retrieval-Augmented Generation for Knowledge-Intensive NLP Tasks.” Advances in Neural Information Processing Systems, 2020.
Share This :