Neuro-Symbolic AI: Enhancing Common Sense in Artificial Intelligence
Artificial Intelligence (AI) is transforming the way we live, work, and interact with technology. From self-driving cars to intelligent chatbots, AI systems are becoming increasingly sophisticated. Yet, despite their impressive capabilities, many AI systems still struggle with something that humans take for granted.
Enter Neuro-Symbolic AI, an innovative hybrid approach that blends neural networks with symbolic reasoning to create more intuitive and intelligent systems. By bridging the gap between data-driven learning and logic-based reasoning, Neuro-Symbolic AI could be the key to unlocking true machine understanding.
What Is Neuro-Symbolic AI?
At its core, Neuro-Symbolic AI is a fusion of two distinct paradigms in artificial intelligence:
- Neural networks, which excel at learning patterns from large datasets through statistical methods.
- Symbolic AI, which uses rules and logic to represent knowledge and reason about the world.
This hybrid approach leverages the strengths of both systems. Neural networks provide flexibility and learning capability, while symbolic reasoning offers structure and interpretability. When combined, they allow AI to learn from raw data while also making logical inferences—an essential trait for achieving common sense (Marcus and Davis 59).
For instance, a traditional neural network might be able to identify objects in a picture, but it won’t understand the relationships between them. Neuro-Symbolic AI, on the other hand, can recognize the objects and also infer that “a cup on a table” implies support from the table—a simple yet profoundly human way of reasoning.
Limitations of Traditional AI
Despite their advancements, traditional deep learning systems are often data-hungry and opaque. These models perform well in narrow, well-defined tasks but lack flexibility when encountering new or ambiguous scenarios.
Common issues include:
- Misinterpreting visual scenes due to missing contextual understanding.
- Failing to solve tasks that require step-by-step logic or multi-step reasoning.
- Inability to generalize knowledge beyond training data (Lake et al. 443).
These shortcomings stem from the fact that deep learning models do not inherently “understand” their environment—they only mimic patterns from data. As a result, they often falter in situations that require reasoning, abstraction, or prior knowledge.
How Neuro-Symbolic AI Works
The workflow of a Neuro-Symbolic AI system typically involves two main stages:
- Perception: A neural network processes raw data—images, text, or audio—and extracts relevant features.
- Reasoning: A symbolic module applies logic and structured knowledge to interpret the results and make decisions.
This combination creates a feedback loop where symbolic reasoning can guide neural learning, and vice versa (Besold et al. 26). For example, in an autonomous vehicle, the neural system might detect road signs and pedestrians, while the symbolic module ensures that decisions follow traffic laws and safety rules.
Applications of Neuro-Symbolic AI
Neuro-Symbolic AI is not just a theoretical concept—it is already showing promise in multiple industries:
- Robotics: Robots equipped with Neuro-Symbolic AI can understand and manipulate objects in a more human-like way, enabling safer and more efficient interactions in dynamic environments.
- Healthcare: By combining data-driven diagnostics with reasoning over symptoms and medical knowledge, AI systems can offer more accurate and explainable diagnoses (Gunning et al. 2).
- Education: Intelligent tutoring systems can better adapt to students’ learning styles by combining behavioral data with educational principles and logic.
These applications demonstrate how blending neural learning with symbolic reasoning creates AI systems that are not only smarter but also more reliable and context-aware.
The Future of Neuro-Symbolic AI
As AI continues to evolve, Neuro-Symbolic AI stands out as a critical advancement in the quest for machine common sense.
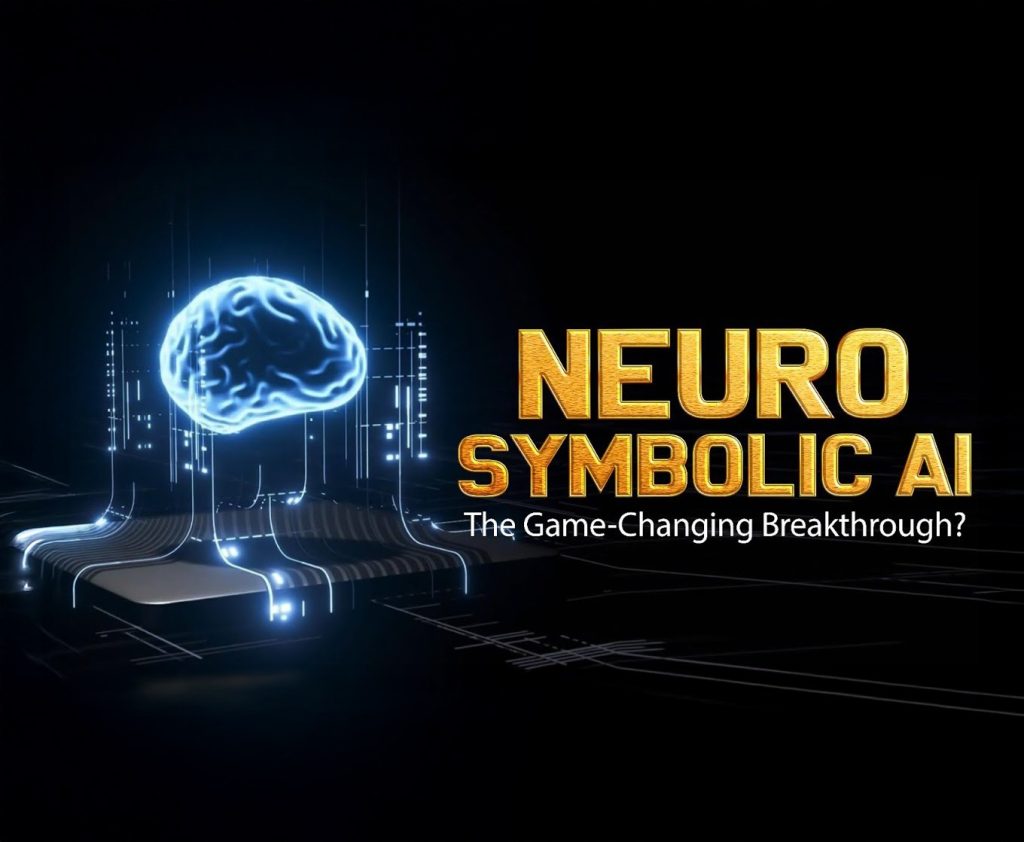
Researchers believe this approach can pave the way for AI systems that understand, reason, and adapt like humans—ushering in a new era of trustworthy and interpretable artificial intelligence (Marcus 31).
From enabling smarter personal assistants to supporting life-saving decisions in medicine, the future of AI may well depend on the success of this hybrid model.
Neuro-Symbolic AI offers a promising path forward by integrating the learning prowess of neural networks with the reasoning capabilities of symbolic logic. This powerful combination can help AI overcome its current limitations and move closer to human-like intelligence.
As we look to the future, this technology could revolutionize fields from robotics and education to healthcare and beyond—ultimately creating systems that not only see and predict but also understand and reason.
Large AI models represent a monumental leap forward in economic forecasting. Their ability to interpret vast data landscapes, anticipate shocks, and suggest proactive strategies could lead to a more stable, resilient, and inclusive global economy. The transformation is underway—and it’s up to us to shape it responsibly.
For further assistance, visit our channel and refer the video
🧠Neuro-Symbolic AI: Could This Be the Breakthrough We Have Been Waiting For? 🧠
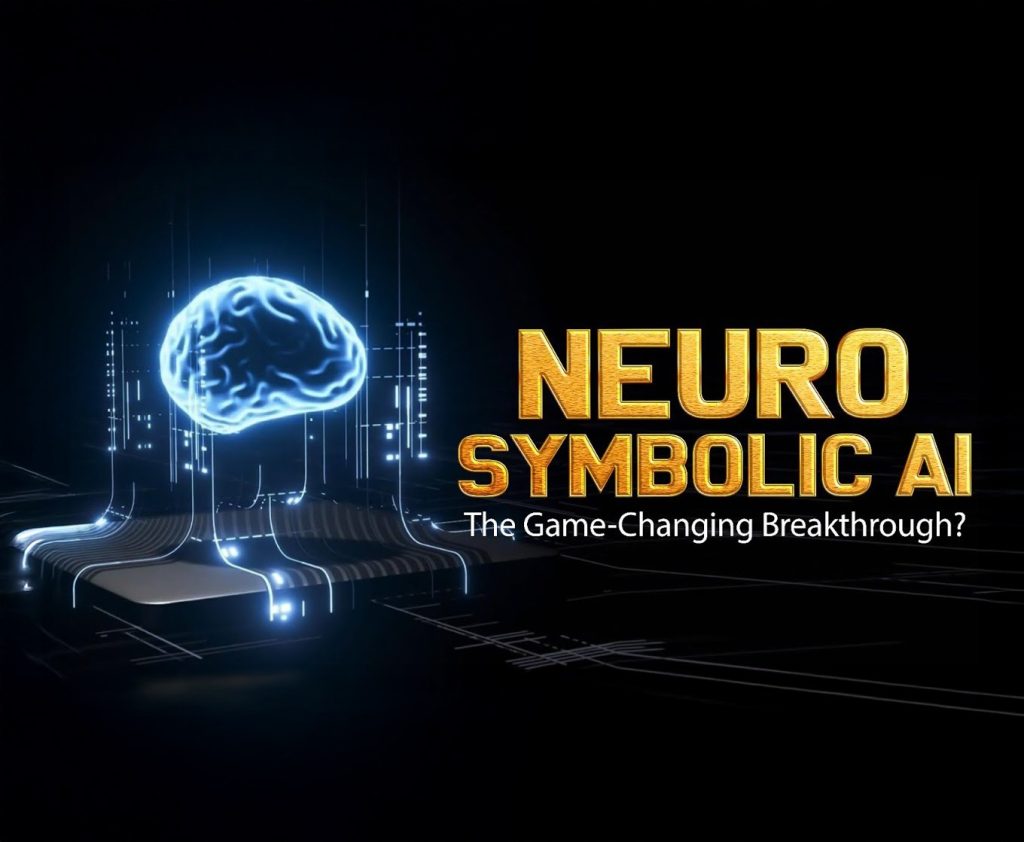
References
- Besold, Tarek R., et al. “Neural-Symbolic Learning and Reasoning: A Survey and Interpretation.” Frontiers in Robotics and AI, vol. 3, 2017, pp. 1–19. DOI:10.3389/frobt.2017.00001.
- Gunning, David, et al. “XAI—Explainable Artificial Intelligence.” Defense Advanced Research Projects Agency (DARPA), 2019, https://www.darpa.mil/program/explainable-artificial-intelligence.
- Lake, Brenden M., et al. “Building Machines That Learn and Think Like People.” Behavioral and Brain Sciences, vol. 40, 2017, pp. 1–58. DOI:10.1017/S0140525X16001837.
- Marcus, Gary, and Ernest Davis. Rebooting AI: Building Artificial Intelligence We Can Trust. Pantheon Books, 2019.
- Marcus, Gary. “The Next Decade in AI: Four Steps Towards Robust Artificial Intelligence.” arXiv preprint, 2020. arXiv:2002.06177.
Share This :